In today’s data-driven environments, large volumes of information flow through complex networks of systems and tools. Data pipeline orchestration refers to the automated coordination and management of these data flows to ensure they run reliably, efficiently, and at scale.
If you’re new to the concept, start with our complete Data Orchestration Guide. For more nuanced discussions, check out data orchestration vs ETL and data pipeline orchestration comparisons.
Defining Data Pipeline Orchestration.
A data pipeline comprises multiple steps: extraction of raw data, transformation into analytics-ready formats, loading into target systems (data warehouses, lakes, or dashboards), and potentially applying machine learning models or data quality checks.
Orchestration is the ‘conductor’ ensuring these steps occur in the right sequence, with the right dependencies, and at the right times. It involves:
- Scheduling tasks and workflows.
- Managing dependencies and conditional logic.
- Monitoring performance, failures, and retries.
- Handling resource allocation and cost optimisation.
Key Components of Data Pipeline Orchestration:
- Workflow Definition: Pipelines are often defined as Directed Acyclic Graphs (DAGs), where each node represents a task, and edges represent dependencies.
- Scheduling and Triggers: Orchestration tools support time-based schedules (e.g., nightly runs), event-driven triggers (e.g., new data arrival), or condition-based triggers (e.g., when a certain metric exceeds a threshold).
- Error Handling and Alerts: Automatic retries, notifications, and escalation protocols ensure minimal downtime.
- Integration with Various Technologies: Pipelines may involve multiple storage systems (AWS S3, Azure Blob Storage), processing engines (Spark, Flink), or ML frameworks (TensorFlow, PyTorch). Orchestration tools must integrate seamlessly with these technologies.
Why is Data Pipeline Orchestration Important?
- Scalability: Orchestration helps manage growing data volumes and complexity without manual intervention.
- Reliability: Automated error handling and dependency checks reduce the risk of pipeline failures.
- Performance Optimisation: Dynamic resource allocation and workload balancing ensure cost-effective, high-throughput operations.
- Compliance and Governance: By enforcing structured workflows and logging lineage data, orchestration supports compliance with local and international regulations, including those in Australia.
Use Cases and Examples:
- Cloud Migration: As organisations shift from on-prem to cloud, data pipeline orchestration tools help maintain business continuity and data integrity.
- Real-Time Analytics: Streaming data from IoT sensors or social media feeds can be orchestrated for instant insights and anomaly detection.
- Machine Learning Lifecycles: Orchestration ensures model retraining, testing, and deployment occur seamlessly in response to data changes.
For more illustrative scenarios, review our examples of data orchestration.
Choosing the Right Tool + Future Directions
Tools like Apache Airflow, Prefect, and managed cloud services (AWS Step Functions, GCP Cloud Composer) each offer unique capabilities. Your selection depends on your technology stack, compliance requirements, and workload types. To explore which solutions might fit best, read our article on the best data orchestration tools.
As we move into the future, expect advanced features like AI-driven pipeline optimisation, better support for hybrid and multi-cloud environments, and closer integration with ML and BI platforms. As global data footprints expand, orchestration will become even more critical, ensuring that regional compliance needs are met without sacrificing agility.
Conclusion.
Data pipeline orchestration is the backbone of modern data engineering. By automating workflows and integrating with various technologies, it enables reliable, scalable, and cost-effective data operations.
To complement orchestration with world-class analytics, ML, GenAI, and custom app creation, consider our Rayven Platform. With Rayven, you can streamline orchestration while simultaneously tapping into the entire spectrum of advanced data capabilities.
Author
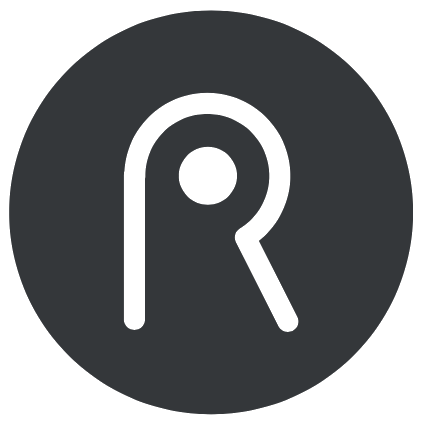