In today's modern business environments, remote real-time asset monitoring can be critical to the success of an industrial organization, and IoT and AI technology have revolutionized the way asset monitoring is performed, making it more efficient and effective than ever before.
When being used for real-time asset monitoring, these technologies are often combined in a single pIoT platform that gives organizations and their employees the ability to monitor assets' real-time performance via dashboards remotely and apply machine learning algorithms or custom business logic to deliver on a range of all-new capabilities for improving performance, maintenance and other benefits.
However, in order to do this, they must have ready access to real-time, accurate data.
The role of data ETL in real-time asset monitoring
To utilize IoT and AI technology for real-time asset monitoring, data streams must first be reliably and consistently collected and collated from IoT sensors, machinery software, and other related systems; before being transformed into a usable format. This process is known as ETL (Extract, Transform, Load), which involves extracting data from multiple sources, transforming the data into a standardized format, and loading the data into a data warehouse or other data storage system for analysis (by the platform).
This is by far and away the most challenging aspect of delivering on any real-time asset monitoring project, however it's critical, as only once all relevant data is being collected and transformed through the ETL process on an ongoing basis can it be used to populate dashboards for personnel or be leveraged by machine learning algorithms to deliver predictive insights into assets' health and performance.
Advanced industrial platforms, such as Rayven Dynamix, are able to combine the data, AI + IoT elements in a single end-to-end platform. Dynamix features unrivalled data integration and interoperability capabilities, meaning the data element of running an Industry 4.0 platform can be carried out in the space place and the analysis and optimization elements. The advantage of this is that, not only do you have the costs of running a single system, but you have significantly reduced complexity originating from multiple integrations demanding real-time data processing.
The benefits of using an end-to-end data, AI + IoT platform for real-time asset monitoring.
Real-time monitoring of assets, predictive maintenance, data anomaly detection, and improved data analytics are some of the ways that integrated data, AI + IoT technology can be used to improve asset monitoring.
Real-time asset monitoring: Real-time monitoring of assets is one of the significant benefits of IoT and AI technology. IoT sensors can be placed on assets to track their location, usage, and performance. This data is then transmitted to a central system through a data pipeline that uses ETL processes to transform the data into a usable format. The transformed data is then analyzed using AI algorithms to provide real-time insights into the asset's health and performance.
For example, a company can use IoT sensors to monitor the performance of their machinery. The sensors can track the machine's temperature, speed, and vibration levels. If any of these metrics fall outside the normal range, the system can alert maintenance personnel to investigate the issue. This proactive approach can help prevent equipment failure and reduce downtime, leading to significant cost savings for the company.
Predictive Maintenance (PdM): Predictive maintenance involves using data and analytics to predict when maintenance is needed before equipment failure occurs. This approach can help companies avoid unplanned downtime, reduce maintenance costs, and extend the lifespan of their assets.
To implement predictive maintenance, IoT sensors are used to monitor the wear and tear on equipment. The data is collected through a data pipeline and transformed using ETL processes. The transformed data is then analyzed using AI algorithms to predict when maintenance is needed. The AI system can alert maintenance personnel to schedule maintenance before a failure occurs, preventing downtime and reducing repair costs.
Data anomaly detection: Data anomaly detection involves identifying unusual patterns or outliers in data that may indicate a problem or a performance issue. For example, if the temperature of a machine suddenly spikes, it may indicate a malfunction. Anomaly detection can help companies quickly identify and address issues before they become serious problems.
To detect anomalies in data, IoT sensors collect data that is transformed using ETL processes before being analyzed using AI algorithms or personnel. By detecting issues early, this can prevent unnecessary downtime and ensure that output and uptime is maximized.
Data analytics: Improved data analytics is also a significant benefit of IoT and AI technology. By collecting data from IoT sensors, companies can gain insights into their asset performance and usage patterns. This enables personnel (or machine learning algorithms) to analyze this data to compare and contrast the performance of different assets or fleets, identifying trends, anomalies, and areas for improvement, as well as provide insights that might direct future maintenance or purchasing decisions.
To improve data analytics, the data collected through IoT sensors must be transformed using ETL processes and loaded into a data warehouse or other data storage system. This allows for easy analysis and visualization of the data. For example, a logistics company may use IoT sensors to track the location and condition of their vehicles. The data collected from these sensors can be transformed using ETL processes and loaded into a data warehouse. AI algorithms can then be used to analyze the data and identify trends in vehicle usage, such as which routes are most frequently used or which vehicles require more maintenance. This information can be used to optimize fleet operations and reduce costs.
In conclusion, integrated data, AI + IoT technology can greatly improve asset monitoring in a variety of ways, with real-time monitoring of assets, predictive maintenance, data anomaly detection, and improved data analytics being just a few of the benefits of this technology. However, to effectively deliver on these, data must first be collected and collated from IoT sensors, machine software and other sources before being transformed into a usable format through the ETL process.
To discover more about how our Rayven Dynamix integrated data, IA + IoT platform can achieve this for you or to explore our ready-to-use real-time asset monitoring IoT solutions built for industry, speak to us today.
Author
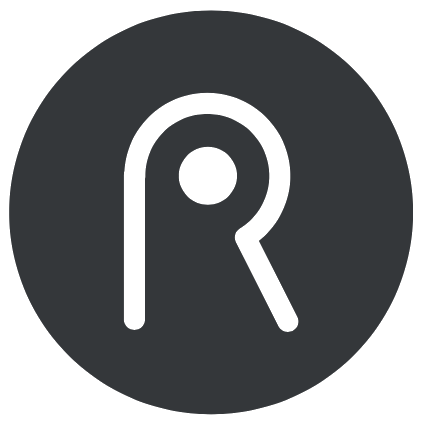